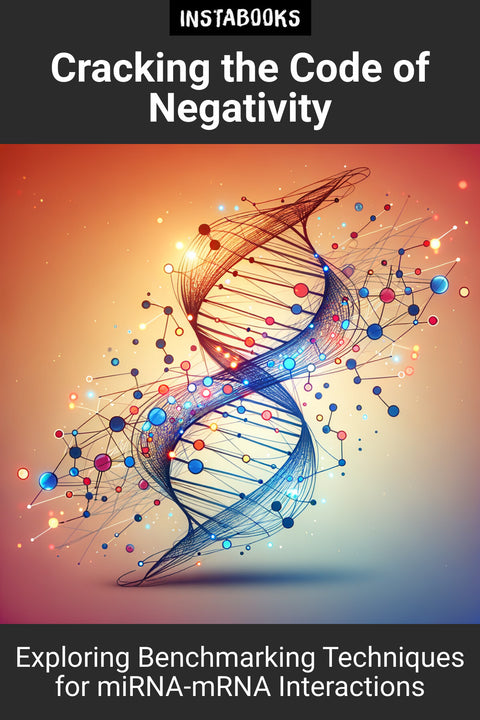
Instabooks AI (AI Author)
Cracking the Code of Negativity
Exploring Benchmarking Techniques for miRNA-mRNA Interactions
Premium AI Book (PDF/ePub) - 200+ pages
Introduction
The world of miRNA-mRNA interactions is deeply complex, requiring precise classification for accurate post-transcriptional gene regulation. But what if our methods for generating data are flawed? In "Cracking the Code of Negativity," we delve into the impactful world of negative data generation and benchmarking in miRNA-mRNA interaction classification. This book provides a groundbreaking exploration into how negative datasets can significantly skew results if not properly standardized and evaluated.
Generating Negative Interactions
Understanding negative data generation is crucial for accurate classification. From mock miRNA through sequence shuffling to employing extensive TarBase and CLIP datasets, we detail multiple modern methodologies. Each chapter breaks down a method with comprehensive pseudocode, implementation strategies, and the pros and cons inherent in each approach.
Evaluating Performance: Intra vs. Cross-Dataset Analysis
In the domain of machine learning, performance evaluation is pivotal. We explain the nuances of intra-dataset analysis, examining model effectiveness within a single dataset, as well as cross-dataset analysis, comparing myriad datasets. Discover how nuanced changes in methodology can drastically affect model generalization and accuracy.
Standardization: The Key to Reliable Results
Lack of standardized methods leads to inconsistencies in results, making cross-study comparison arduous. We propose innovative strategies for establishing standard procedures, ensuring reliability and enhancing the comparability of miRNA-mRNA interaction models globally. This book emphasizes the urgency of uniformity in scientific methodologies.
Future Directions and Real-World Applications
Through detailed case studies and applications, "Cracking the Code of Negativity" presents real-world implications and future challenges in miRNA-mRNA classification. Discover emerging trends and future directions poised to enhance model accuracy, preparing you for the next wave of genetic interactions research.
Table of Contents
1. Introduction to miRNA-mRNA Interactions- Role of miRNAs
- Gene Regulation Dynamics
- Importance of Accurate Classification
2. Methods for Generating Negative Interactions
- Mock miRNA Techniques
- Utilizing TarBase Data
- Complementary CLIP Data
3. Performance Evaluation Techniques
- Intra-Dataset Analysis
- Cross-Dataset Comparison
- Effectiveness Measures
4. Impact on Model Accuracy
- Case Study Analysis
- Bias Mitigation Strategies
- Generalization Challenges
5. Standardization and Comparison
- Need for Uniform Methods
- Strategies for Standardization
- Enhancing Cross-Study Comparability
6. Case Studies and Applications
- Real-World Implications
- Success Stories
- Implementing Standard Methods
7. Future Directions and Challenges
- Emerging Trends
- Research Directions
- Overcoming Challenges
8. Role of Negative Data in Machine Learning
- Significance in Model Training
- Impact on Accuracy
- Long-term Outcomes
9. Exploring Data Diversity
- Diversity in Data Generation
- Innovation in Methodologies
- Future Perspectives
10. Mitigating Data Generation Bias
- Impact on Results
- Correction Techniques
- Consistent Outcome Strategies
11. Real-World Applications and Future Trends
- Applications in Genetics
- Technological Advancements
- Projected Trends
12. Conclusive Insights and Reflections
- Lessons Learned
- Future Exploration
- Maximizing Potential
Target Audience
Bioinformatics researchers, data scientists, and geneticists interested in miRNA-mRNA interactions and machine learning model optimization.
Key Takeaways
- Understand the importance and challenges of negative data in miRNA-mRNA interaction classification.
- Learn various methods for generating negative interactions and their effects.
- Explore performance evaluation techniques: intra-dataset and cross-dataset analysis.
- Discover the impact of negative data on machine learning model accuracy and generalization.
- Recognize the need for standardized methods to enhance research reliability.
- Apply insights from real-world case studies and future research directions.
How This Book Was Generated
This book is the result of our advanced AI text generator, meticulously crafted to deliver not just information but meaningful insights. By leveraging our AI book generator, cutting-edge models, and real-time research, we ensure each page reflects the most current and reliable knowledge. Our AI processes vast data with unmatched precision, producing over 200 pages of coherent, authoritative content. This isn’t just a collection of facts—it’s a thoughtfully crafted narrative, shaped by our technology, that engages the mind and resonates with the reader, offering a deep, trustworthy exploration of the subject.
Satisfaction Guaranteed: Try It Risk-Free
We invite you to try it out for yourself, backed by our no-questions-asked money-back guarantee. If you're not completely satisfied, we'll refund your purchase—no strings attached.